Numlock Awards: James England's Model
The Numlock Awards Supplement is your one-stop awards season update. You’ll get two editions per week, one from Not Her Again’s Michael Domanico breaking down an Oscar contender or campaigner and taking you behind the storylines, and the other from Walt Hickey looking at the numerical analysis of the Oscars and the quest to predict them. Today’s guest column comes from our friend James England.
Hey Y’all!
Welcome to a very special Friday edition of the Numlock Awards Supplement. I'm James England, your guest host for the day. For those of you who have followed Walt's coverage of the Oscars over the years, you may recall that he ran a "bake-off" of amateur algorithms leading up to the 2016 Academy Awards. I submitted an idea I'd been playing with to convert my College Football Rankings algorithm into something reusable for more than just sports and the idea of the Oscar Ratings was born. That first year, the ratings selected Spotlight as Best Picture. The following year, in perhaps my greatest accomplishment to date, the ratings nailed Moonlight. This hot streak did unfortunately come to an end last year when The Shape of Water, a film about a lady and a fish bonding over what must have been some terrific hard-boiled eggs, took out ratings favorite Lady Bird. In an attempt to right that ship, I'm back again this year looking to predict Best Picture, as well as some of the other top awards for the evening.
Now that we're all acquainted, I'd like to take you through the process of how these ratings work.
College Football, Huh?
Before we get to the silver screen, let's go to the gridiron. Back in 2012, my beloved Alma Mater, Utah State University (Go Aggies), was having a historic football season. As I'm sure you all recall, they finished that season 11-2 and ranked #16 in the AP poll. This was not something I was used to, having watched them win a total of 9 games over the 4 years I was a student. In what I can only assume is a typical reaction to this newfound success, as the season was progressing I decided that I wanted to build my own ratings system, similar to the BCS computer algorithms that were being used to determine who would play in the National Championship game. The system I ended up using is a Linear Regression Model. It's a great system for college football because it allows you to get a pretty good comparison of all teams when most of them never play against each other.
How Do Football Ratings Translate to the Oscars?
I'm glad you asked! Building a linear regression model with a ton of data points can be complicated, but at the heart of what I'm trying to do is take a list of games played, determine the winners, and assign a score difference for each game. In football, that can be visualized like this:

In the above figure, 1 signifies the team that won, -1 signifies the team that lost and 0 is a placeholder for a team that wasn't participating in the game. Once you add up enough of these data points relative to the amount of teams in your league, you can begin to assess actual quality of a team compared to another using some pretty complex math involving matrices. When that process is completed, each team would be given a weighted score indicating their overall quality to-date. That score can then help us determine if the Bears are a better team than the Scarecrows even if they never played head-to-head.
Now let's say that we wanted to pull the context of football completely out of this process. What if instead we set up a simple webpage that asked a person which movies they saw this year and then follow up with questions to compare those movies, directors, actors and actresses against each other head-to-head?

It's basically the same thing! Each game is now a result of one movie against the other, with the score difference always set at 1, which really just makes the math easier. In the figure above, someone liked Roma more than A Star is Born, A Star is Born more than Black Panther, and Green Book more than Roma. That person doesn't have to figure out if that means that Green Book is their choice for Best Picture, just that they liked it more than Roma and then moved on to the next question. I can then ask these types of questions to as many people on the internet as possible and start to get some really interesting information.
The core idea for this experiment to work is that the type of person who takes the time to watch a handful of these types of movies and then vote on them on a random person's personal website is just about as good at determining “which is better” as someone with an actual ballot for the awards.
This is a bit of a leap of faith, but I don't think it's a completely crazy idea... and that's exactly what the Oscar Ratings are doing. I have been asking people to go to my website and choose head-to-head winners for the following categories: Best Picture, Best Director, Best Actress, Best Actor, Best Supporting Actress and Best Supporting Actor. These matchup results are then combined together, placed into my open source ranking methods and the result are a series of scores for each category.
Determining The Winners
Once I run the ratings, we aren't quite done. When testing the initial model, there was one glaring issue - how many people actually watched some of these movies can make a big difference when it comes to the results in real life. If a nominee has a terrific score, but a very low amount of actual matchups, it gets knocked down a little bit. A good example of the need for this was last year's Best Supporting Actor race.
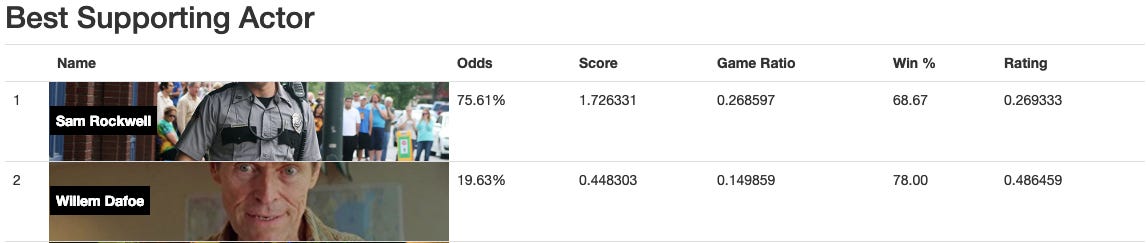
Sam Rockwell was accurately predicted to win this award, but if we had only evaluated this race by the raw Rating number, Willem Dafoe should have been comfortably ahead. The problem for Willem was a lot more people saw Three Billboards than The Florida Project and that has to be accounted for. The Game Ratio value tells us, compared to the other nominees in the category, how frequently this nominee was voted on. In this instance that means that a voter was almost twice as likely to have seen Rockwell's performance (.268) than Dafoe's (.149). This means that the final step is to weight the Rating, Game Ratio and Win % values and come up with the final Score that will determine the prediction.
What About This Year?
You've made it this far, it's time for the good stuff. Let's take a look at some results.
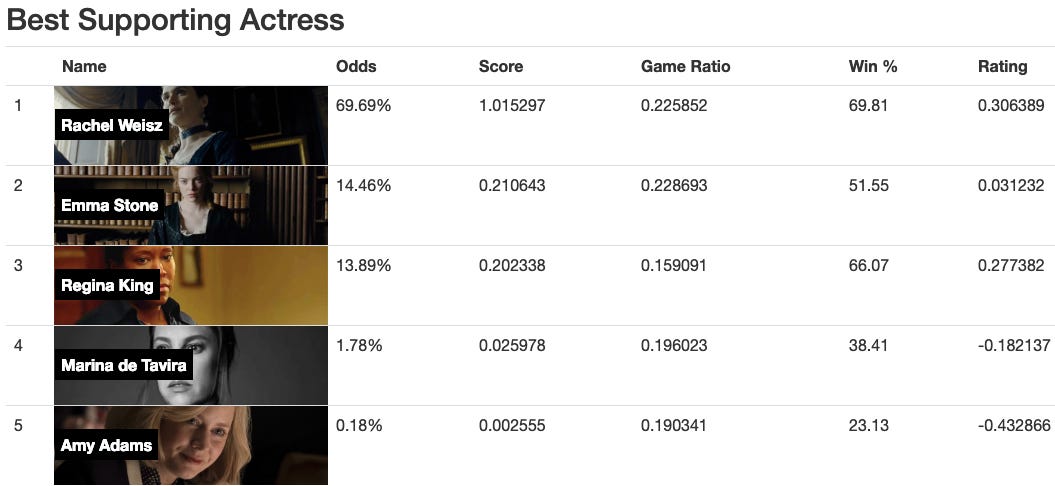
A very interesting category this year! Rachel Weisz and Emma Stone are currently sitting in the #1 and #2 spots. You can see that since they're both nominated for their roles in The Favourite that their Game Ratios are essentially the same. Regina King actually out-rated Stone, but couldn't make up enough of the viewership deficit to surpass her on the odds. Prediction: Rachel Weisz.
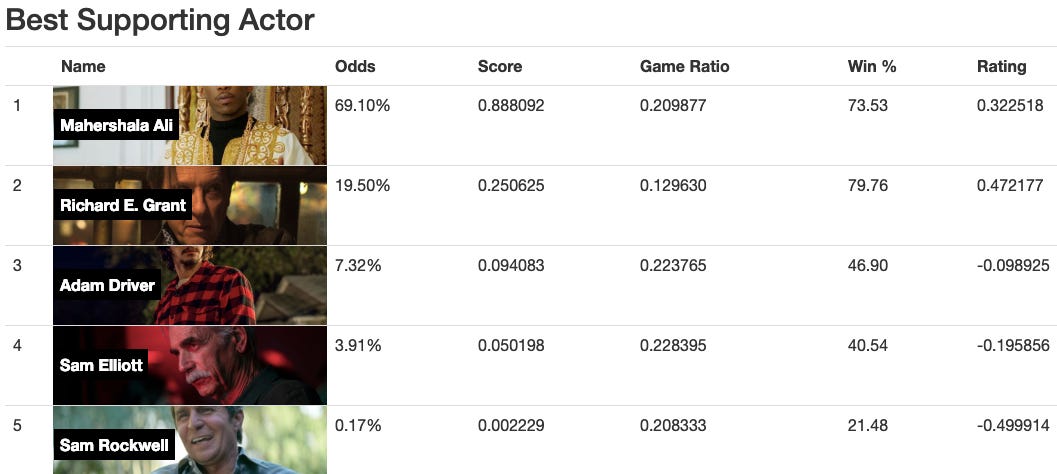
This looks to be a two-man race between Mahershala Ali and Richard E. Grant. Ali's strong Game Ratio and comparable Win % and Rating give him the nod. Prediction: Mahershala Ali.
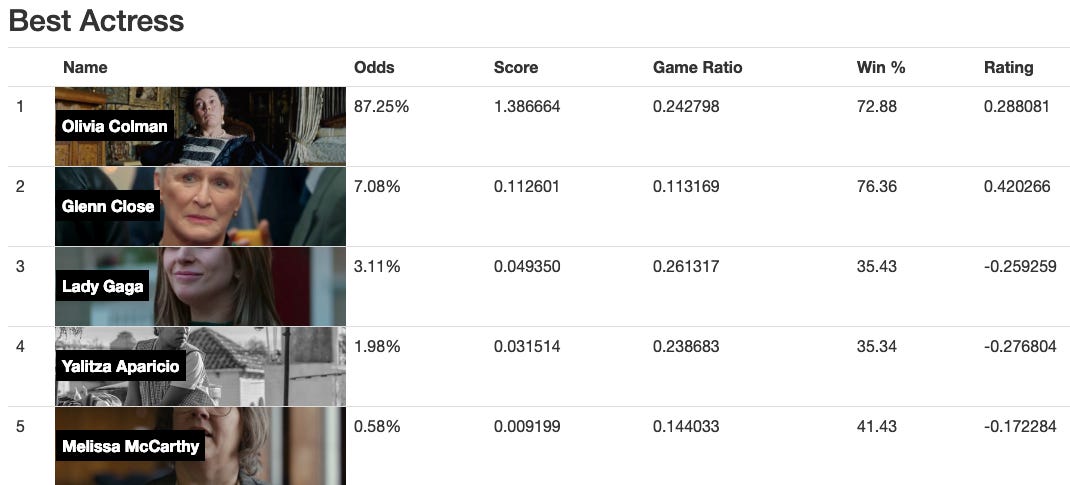
The Rating score clearly shows that this is a race between Olivia Colman and Glenn Close. Colman is given the edge based on a much stronger Game Ratio, but both she and Close have very good Ratings. If more members of the Academy popped in the screener for The Wife than the population voting on my site (or just took a friend's word for it and put her name on the top of the ballot), these odds could very well be off. Prediction: Olivia Colman.
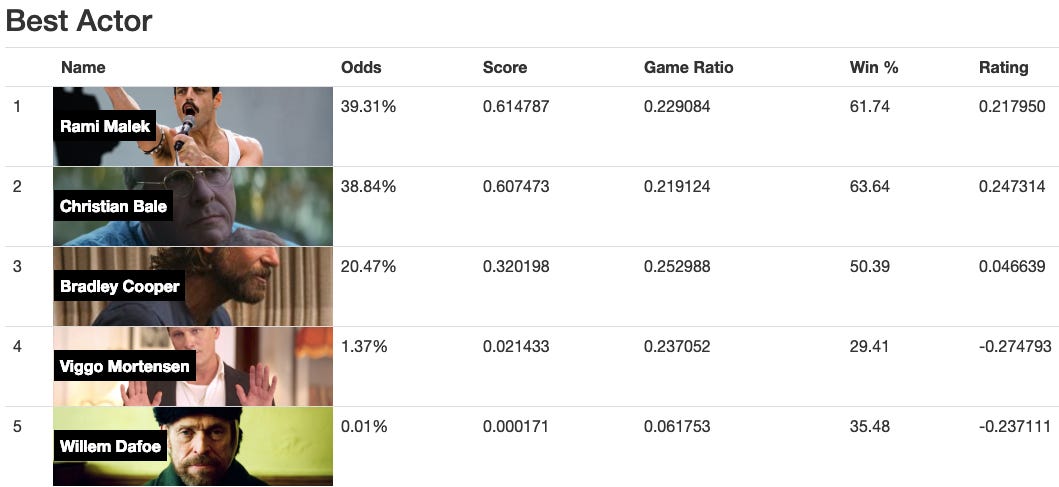
Flip a coin. Basically every metric on here is interchangeable between Rami Malek and Christian Bale. Bradley Cooper also has a not-so-bad outside chance, but this one really looks to be shaping up into a photo-finish between Freddie Mercury and Dick Cheney. Prediction: Rami Malek.
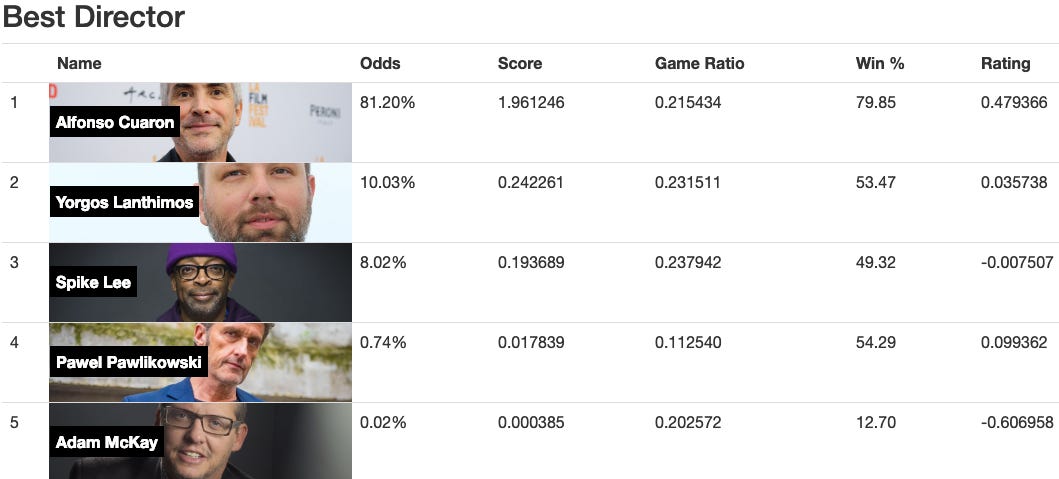
Alfonso Cuarón is crushing this one. It sure looks like Spike Lee's going to need to wait for another year to get his statue, but it is an honor just to be nominated. Prediction: Alfonso Cuarón.
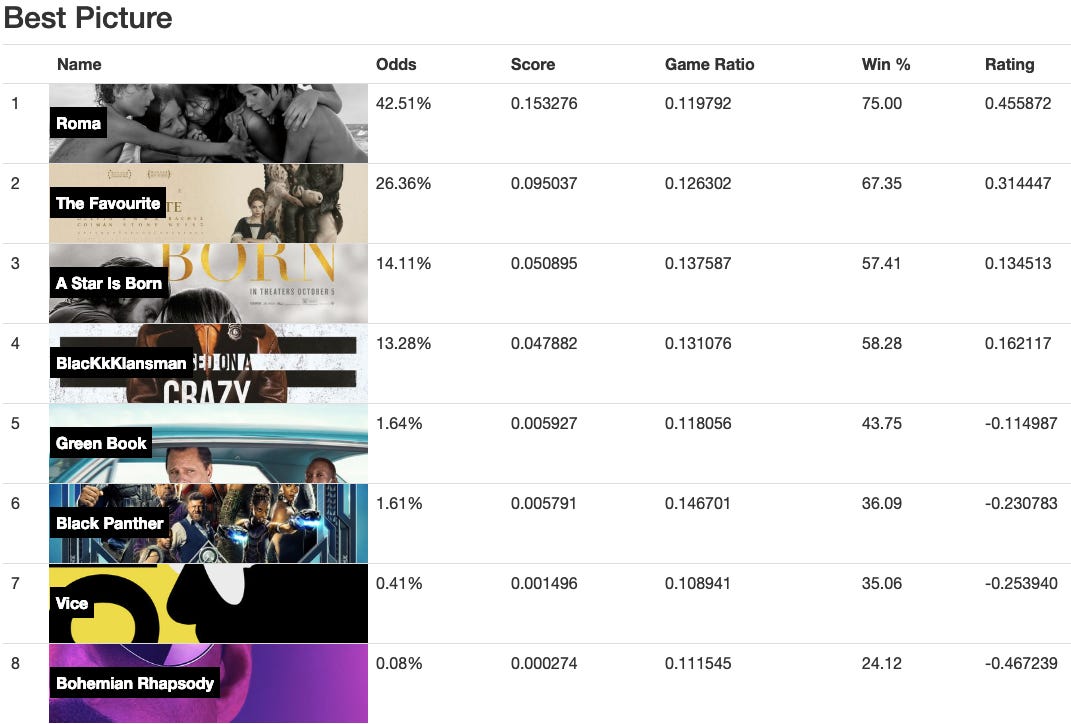
The Game Ratios in this Best Picture race are insanely even, so that becomes pretty much a non-factor in this year's race. The big surprise in this category isn't necessarily which film is on top, but what is sitting at the #2 spot. Based on these ratings, it would not be out of the realm of possibility for The Favourite, which I believe is sitting at last place in Walt's simulation, pulled off an upset on Sunday. Prediction: Roma.
There you have it! Thank you so much to all of you who have participated by voting on my site. The poll will still be available through Saturday, so it isn't too late for you to mess up all of these predictions that I've typed up. Many thanks also to Walter and Michael for inviting me to hop on their platform and talk about all this. If you're so inclined, you can find me online @JEinOKC on most social platforms. I am terrible at taking constructive criticism from strangers, but am very welcoming of new followers and unsolicited flattery.